I am going to tell you why I don’t think you should sign your Git commits, even
though doing so with SSH keys is now easier than ever. But first, to
contextualize my objection, I have a brief hypothetical for you, and then a bit
of history from the evolution of security on the web.
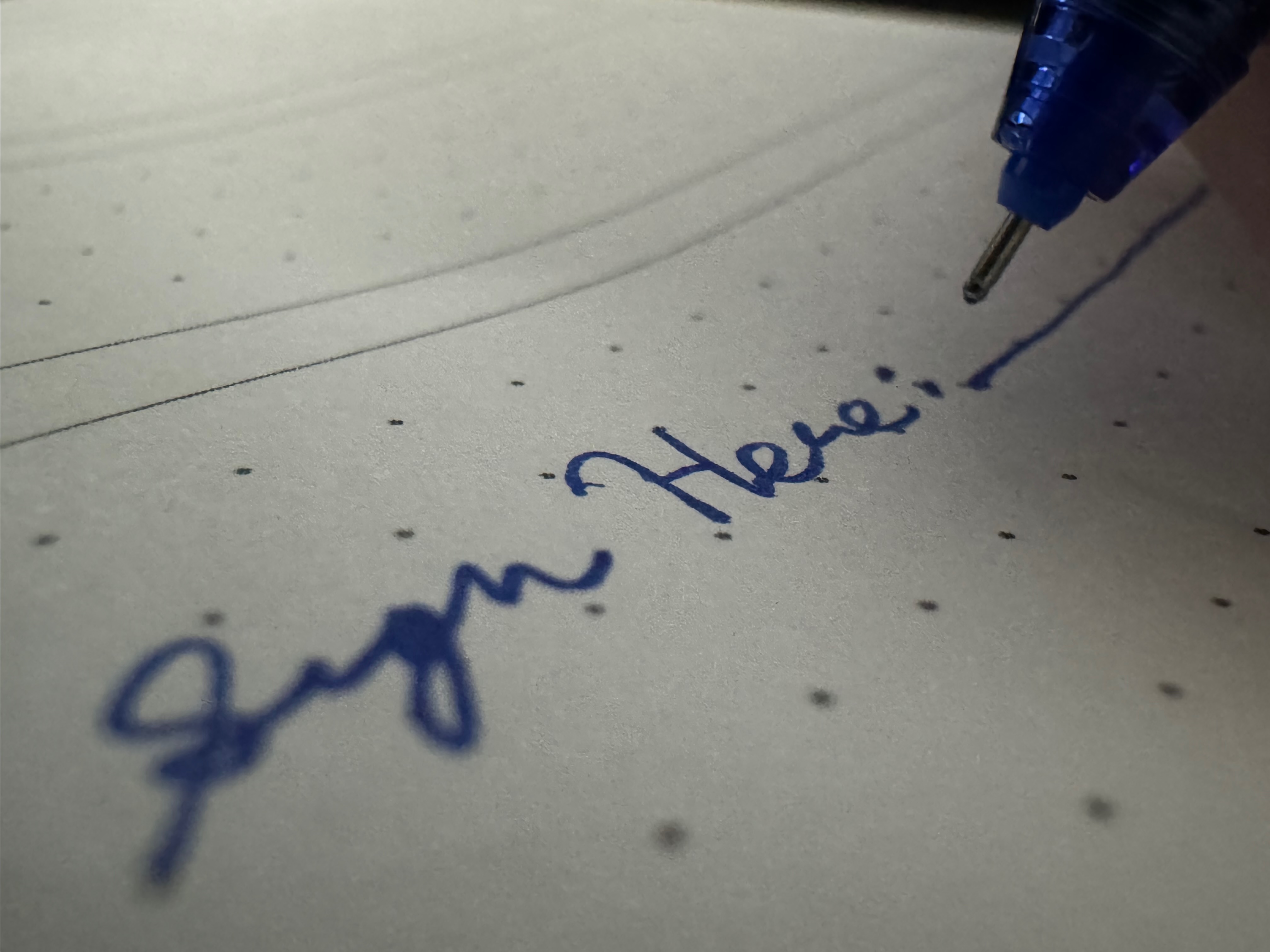
It seems like these days, everybody’s signing all different kinds of papers.
Bank forms, permission slips, power of attorney; it seems like if you want to
securely validate a document, you’ve gotta sign it.
So I have invented a machine that automatically signs every document on your
desk, just in case it needs your signature. Signing is good for security, so
you should probably get one, and turn it on, just in case something needs your
signature on it.
We also want to make sure that verifying your signature is easy, so we will
have them all notarized and duplicates stored permanently and publicly for
future reference.
No? Not interested?
Hopefully, that sounded like a silly idea to you.
Most adults in modern civilization have learned that signing your name to a
document has an effect. It is not merely decorative; the words in the
document being signed have some specific meaning and can be enforced against
you.
In some ways the metaphor of “signing” in cryptography is bad. One does not
“sign” things with “keys” in real life. But here, it is spot on: a
cryptographic signature can have an effect.
It should be an input to some software, one that is acted upon. Software
does a thing differently depending on the presence or absence of a signature.
If it doesn’t, the signature probably shouldn’t be there.
Consider the most venerable example of encryption and signing that we all deal
with every day: HTTPS. Many years ago, browsers would happily display
unencrypted web pages. The browser would also encrypt the connection, if the
server operator had paid for an expensive certificate and correctly configured
their server. If that operator messed up the encryption, it would pop up a
helpful dialog box that would tell the user “This website did something wrong
that you cannot possibly understand. Would you like to ignore this and keep
working?” with buttons that said “Yes” and “No”.
Of course, these are not the precise words that were written. The words, as
written, said things about “information you exchange” and “security
certificate” and “certifying authorities” but “Yes” and “No” were the words
that most users read. Predictably, most users just clicked “Yes”.
In the usual case, where users ignored these warnings, it meant that no user
ever got meaningful security from HTTPS. It was a component of the web stack
that did nothing but funnel money into the pockets of certificate authorities
and occasionally present annoying interruptions to users.
In the case where the user carefully read and honored these warnings in the
spirit they were intended, adding any sort of transport security to your
website was a potential liability. If you got everything perfectly correct,
nothing happened except the browser would display a picture of a small green
purse. If
you made any small mistake, it would scare users off and thereby directly harm
your business. You would only want to do it if you were doing something that
put a big enough target on your site that you became unusually interesting to
attackers, or were required to do so by some contractual obligation like credit
card companies.
Keep in mind that the second case here is the best case.
In 2016, the browser makers noticed this problem and started taking some
pretty aggressive
steps
towards actually enforcing the security that HTTPS was supposed to provide, by
fixing the user interface to do the right thing. If your site didn’t have
security, it would be shown as “Not Secure”, a subtle warning that would
gradually escalate in intensity as time went on, correctly incentivizing site
operators to adopt transport security certificates. On the user interface
side, certificate errors would be significantly harder to disregard, making it
so that users who didn’t understand what they were seeing would actually be
stopped from doing the dangerous thing.
Nothing fundamental changed about the technical aspects of the
cryptographic primitives or constructions being used by HTTPS in this time
period, but socially, the meaning of an HTTP server signing and encrypting
its requests changed a lot.
Now, let’s consider signing Git commits.
You may have heard that in some abstract sense you “should” be signing your
commits. GitHub puts a little green “verified” badge next to commits that are
signed, which is neat, I guess. They provide “security”. 1Password provides a
nice UI for
setting it up. If you’re not a 1Password user, GitHub itself recommends you
put in just a few lines of
configuration
to do it with either a GPG, SSH, or even an S/MIME key.
But while GitHub’s documentation quite lucidly tells you how to sign your
commits, its explanation of
why
is somewhat less clear. Their purse is the word “Verified”; it’s still green.
If you enable “vigilant
mode”,
you can make the blank “no verification status” option say “Unverified”, but
not much else changes.
This is like the old-style HTTPS verification “Yes”/“No” dialog, except that
there is not even an interruption to your workflow. They might put the
“Unverified” status on there, but they’ve gone ahead and clicked “Yes” for you.
It is tempting to think that the “HTTPS” metaphor will map neatly onto Git
commit signatures. It was bad when the web wasn’t using HTTPS, and the next
step in that process was for Let’s
Encrypt to come along and for
the browsers to fix their implementations. Getting your certificates properly
set up in the meanwhile and becoming familiar with the tools for properly doing
HTTPS was unambiguously a good thing for an engineer to do. I did, and I’m
quite glad I did so!
However, there is a significant difference: signing and encrypting an HTTPS
request is ephemeral; signing a Git commit is functionally permanent.
This ephemeral nature meant that errors in the early HTTPS landscape were
easily fixable. Earlier I mentioned that there was a time where you might
not want to set up HTTPS on your production web servers, because any small
screw-up would break your site and thereby your business. But if you were
really skilled and you could see the future coming, you could set up
monitoring, avoid these mistakes, and rapidly recover. These mistakes didn’t
need to badly break your site.
We can extend the analogy to HTTPS, but we have to take a detour into one of
the more unpleasant mistakes in HTTPS’s history: HTTP Public Key
Pinning, or “HPKP”.
The idea with HPKP was that you could publish a record in an HTTP header where
your site commits to using certain certificate authorities for a period of
time, where that period of time could be “forever”. Attackers gonna attack,
and attack they
did.
Even without getting attacked, a site could easily commit “HPKP Suicide” where
they would pin the wrong certificate authority with a long timeline, and their
site was effectively gone for every browser that had ever seen those pins. As
a result, after a few years, HPKP was completely removed from all
browsers.
Git commit signing is even worse. With HPKP, you could easily make terrible
mistakes with permanent consequences even though you knew the exact meaning
of the data you were putting into the system at the time you were doing it.
With signed commits, you are saying something permanently, but you don’t really
know what it is that you’re saying.
Today, what is the benefit of signing a Git commit? GitHub might present it
as “Verified”. It’s worth noting that only GitHub will do this, since they
are the root of trust for this signing scheme. So, by signing commits and
registering your keys with GitHub, you are, at best, helping to lock in GitHub
as a permanent piece of infrastructure that is even harder to dislodge because
they are not only where your code is stored, but also the arbiters of whether
or not it is trustworthy.
In the future, what is the possible security benefit? If we all collectively
decide we want Git to be more secure, then we will need to meaningfully treat
signed commits differently from unsigned ones.
There’s a long tail of unsigned commits several billion entries long. And
those are in the permanent record as much as the signed ones are, so future
tooling will have to be able to deal with them. If, as stewards of Git, we
wish to move towards a more secure Git, as the stewards of the web moved
towards a more secure web, we do not have the option that the web did. In
the browser, the meaning of a plain-text HTTP or incorrectly-signed HTTPS site
changed, in order to encourage the site’s operator to change the site to be
HTTPS.
In contrast, the meaning of an unsigned commit cannot change, because there are
zillions of unsigned commits lying around in critical infrastructure and we
need them to remain there. Commits cannot meaningfully be changed to become
signed retroactively. Unlike an online website, they are part of a historical
record, not an operating program. So we cannot establish the difference in
treatment by changing how unsigned commits are treated.
That means that tooling maintainers will need to provide some difference in
behavior that provides some incentive. With HTTPS where the binary choice was
clear: don’t present sites with incorrect, potentially compromised
configurations to users. The question was just how to achieve that. With
Git commits, the difference in treatment of a “trusted” commit is far less
clear.
If you will forgive me a slight straw-man here, one possible naive
interpretation is that a “trusted” signed commit is that it’s OK to run in CI.
Conveniently, it’s not simply “trusted” in a general sense. If you signed it,
it’s trusted to be from you, specifically. Surely it’s fine if we bill the
CI costs for validating the PR that includes that signed commit to your GitHub
account?
Now, someone can piggy-back off a 1-line typo fix that you made on top of an
unsigned commit to some large repo, making you implicitly responsible for
transitively signing all unsigned parent commits, even though you haven’t
looked at any of the code.
Remember, also, that the only central authority that is practically trustable
at this point is your GitHub account. That means that if you are using a
third-party CI system, even if you’re using a third-party Git host, you can
only run “trusted” code if GitHub is online and responding to requests for its
“get me the trusted signing keys for this user” API. This also adds a lot of
value to a GitHub credential breach, strongly motivating attackers to sneakily
attach their own keys to your account so that their commits in unrelated repos
can be “Verified” by you.
Let’s review the pros and cons of turning on commit signing now, before you
know what it is going to be used for:
Pro |
Con |
Green “Verified” badge |
Unknown, possibly unlimited future liability for the consequences of running code in a commit you signed |
|
Further implicitly cementing GitHub as a centralized trust authority in the open source world |
|
Introducing unknown reliability problems into infrastructure that relies on commit signatures |
|
Temporary breach of your GitHub credentials now lead to potentially permanent consequences if someone can smuggle a new trusted key in there |
|
New kinds of ongoing process overhead as commit-signing keys become new permanent load-bearing infrastructure, like “what do I do with expired keys”, “how often should I rotate these”, and so on |
I feel like the “Con” column is coming out ahead.
That probably seemed like increasingly unhinged hyperbole, and it was.
In reality, the consequences are unlikely to be nearly so dramatic. The status
quo has a very high amount of inertia, and probably the “Verified” badge will
remain the only visible difference, except for a few repo-specific esoteric
workflows, like pushing trust verification into offline or sandboxed build
systems. I do still think that there is some potential for nefariousness
around the “unknown and unlimited” dimension of any future plans that might
rely on verifying signed commits, but any flaws are likely to be subtle attack
chains and not anything flashy and obvious.
But I think that one of the biggest problems in information security is a lack
of threat modeling. We
encrypt things, we sign things, we institute rotation
policies and
elaborate useless
rules for passwords, because we are looking for a
“best practice” that is going to save us from having to think about what our
actual security problems are.
I think the actual harm of signing git commits is to perpetuate an engineering
culture of unquestioningly cargo-culting sophisticated and complex tools like
cryptographic signatures into new contexts where they have no use.
Just from a baseline utilitarian philosophical perspective, for a given action
A, all else being equal, it’s always better not to do A, because taking an
action always has some non-zero opportunity cost even if it is just the time
taken to do it. Epsilon cost and zero benefit is still a net harm. This is
even more true in the context of a complex system. Any action taken in
response to a rule in a system is going to interact with all the other rules in
that system. You have to pay complexity-rent on every new rule. So an
apparently-useless embellishment like signing commits can have potentially
far-reaching consequences in the future.
Git commit signing itself is not particularly consequential. I have probably
spent more time writing this blog post than the sum total of all the time
wasted by all programmers configuring their git clients to add useless
signatures; even the relatively modest readership of this blog will likely
transfer more data reading this post than all those signatures will take to
transmit to the various git clients that will read them. If I just convince
you not to sign your commits, I don’t think I’m coming out ahead in the
felicific calculus here.
What I am actually trying to point out here is that it is useful to carefully
consider how to avoid adding junk complexity to your systems. One area where
junk tends to leak in to designs and to cultures particularly easily is in
intimidating subjects like trust and safety, where it is easy to get anxious
and convince ourselves that piling on more stuff is safer than leaving things
simple.
If I can help you avoid adding even a little bit of unnecessary complexity, I
think it will have been well worth the cost of the writing, and the reading.
Acknowledgments
Thank you to my patrons who are
supporting my writing on this blog. If you like what you’ve read here and
you’d like to read more of it, or you’d like to support my various open-source
endeavors, you can support my work as a sponsor! I am also available for
consulting work if you think your organization
could benefit from expertise on topics such as “What else should I not apply a cryptographic signature to?”.